Peacock
Designing an Informed BIN-Based Routing Strategy
June 7, 2024
June 7, 2024

Mina Deegan
Mina Deegan
Mina Deegan
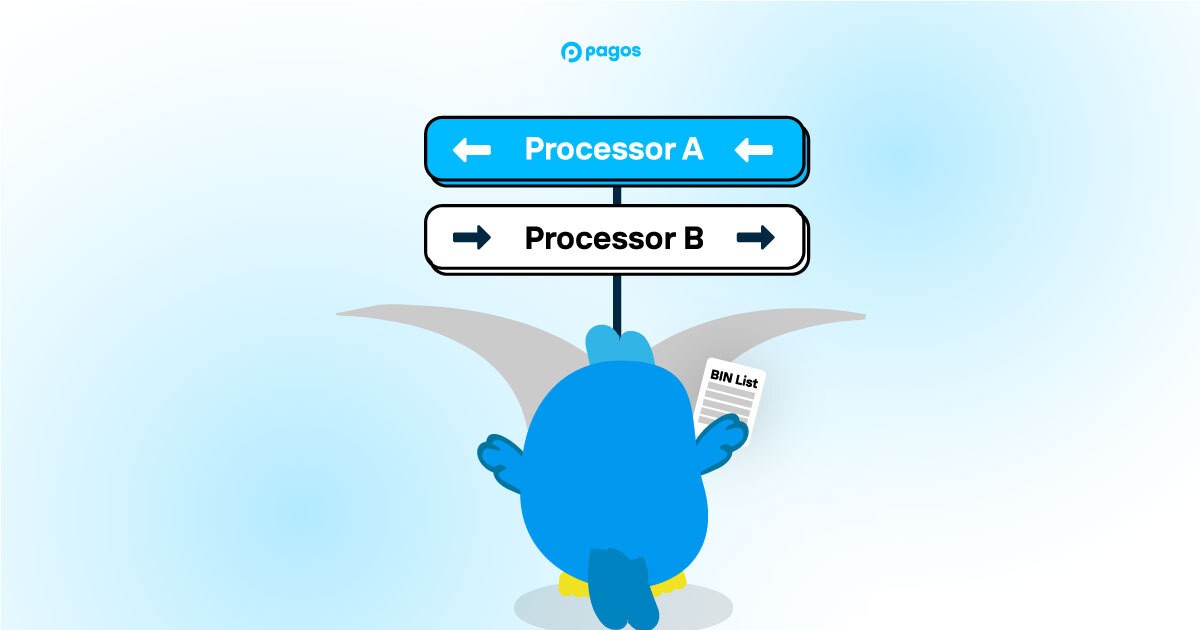
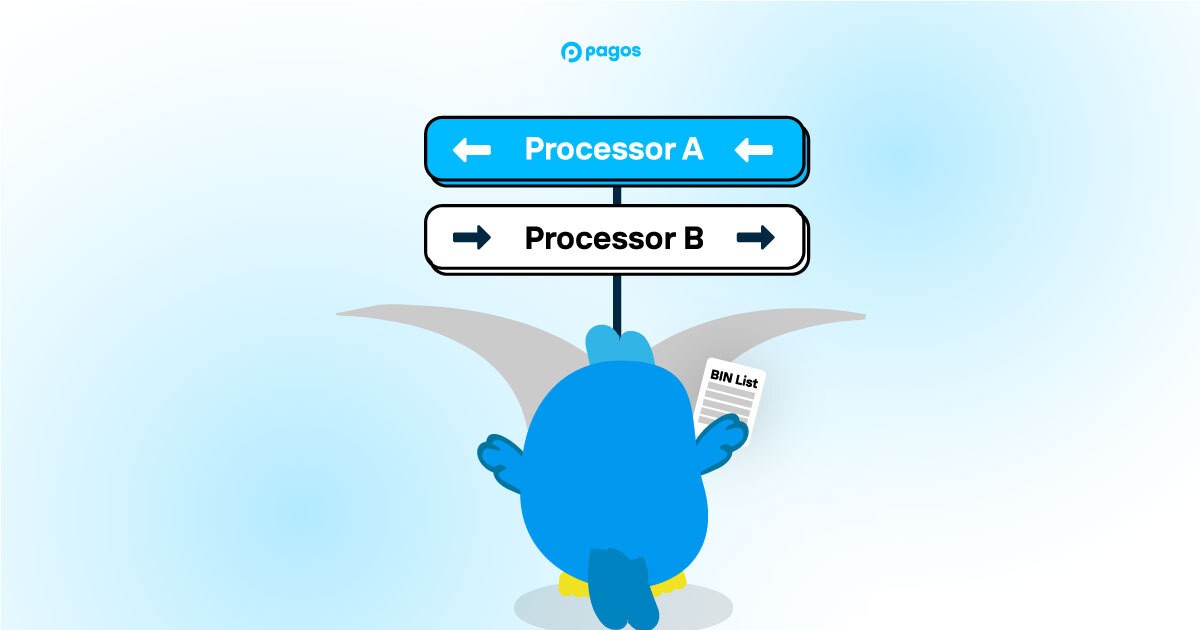
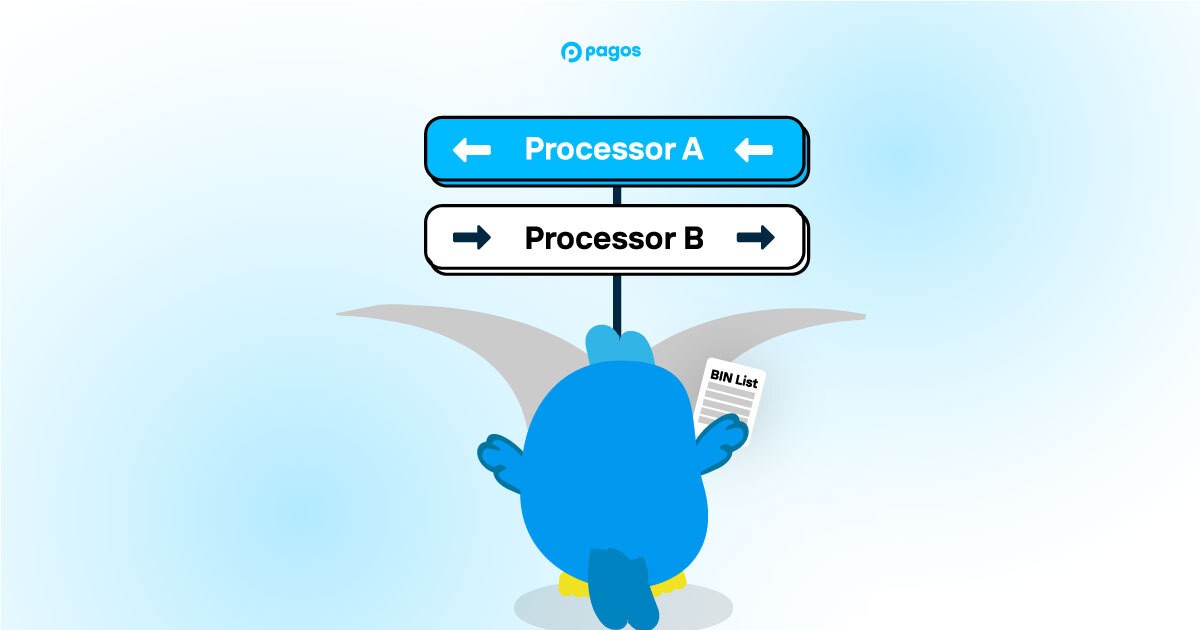
Given the recent explosion in online payments, available payment methods, and payment routing options, companies have a lot to think about when it comes to processing transactions. One such challenge—and opportunity—is deciding which processor to send any given transaction to. While theoretically a payment sent to any processor should see the same result (e.g. success or failure), the complexity of the payments ecosystem and the sheer number of players involved means that’s not always the case. In reality, it’s easy for two processors to diverge in performance due to data quality, compliance updates, fraud rules, integration setup, and even customer mix changes.
In this blog post, we’ll take you through an example of how you can make data-driven decisions around transaction routing using payment card Bank Identification Numbers (BINs). This complete use case explores incorporating BIN data not just into your processing/routing analysis, but into the executed strategy and ongoing monitoring of the results. Being data driven means you should baseline your performance in a normalized way, establish a hypothesis to test, and then track the performance of a given change; in this case we cover routing, but a similar approach can be used for chargeback metrics, cost metrics, and more. Let’s get started!
Note: While this example was written using real data, all data and BINs have been changed or anonymized for the purpose of this story. You can also assume here that the transaction volume distributed across the two processors and BIN is sufficient for a comparison, and that other related metrics like chargeback rates and refund rates are sufficiently similar.
Why BINs?
In previous blog posts, we’ve discussed the importance of integrating bank identification numbers (BINs) into your payments analysis, even going so far as to cite BIN data as a key part of any payments optimization strategy. When it comes to learning who your customers are, where they’re located, and what their preferences are, you can’t do much better than BIN data. Similarly, BINs allow you to divide your customer base up into logical segments for making informed decisions around processing, fraud analysis, tokenization, and more. In this post, we’ll use BINs as a segmentation tool for transaction routing.
The BIN-Based Analysis
To make this post more fun, we’re going to put you in the role of the payments manager at a mid-sized business. Imagine your business processes transactions in the United States through two processors. Your current routing strategy is to route roughly 25% of your transactions through Processor A and 75% through Processor B. Your overall approval rate across all your card transaction volume is fair—around 86%—but you want to improve it to increase your revenue. You’re specifically interested in how you might be able to increase your overall approval rate by being more strategic with your transaction routing.
To better understand your approval rate (and how to improve it), you start a deep dive analysis with Pagos. Because Pagos ingests all your data from both your processors and aggregates it together into a single, harmonized data set, you can see all your transaction data in one place, broken down by whatever parameters matter to you most. For this particular investigation, you’re interested in seeing your approval rate broken down by BIN for both processors. In other words, you want to see if some BINs perform better with one payment processor over the other; if so, you can design routing rules to send transactions made with certain BIN ranges to the processor where it’s most likely to succeed!
For this analysis, you filter your data from the last six months to only see transactions routed through Processor A, broken down by BIN. And for each BIN, you want to see attempted vs. approved transaction counts and value, alongside the approval rate. You do the same for transactions from Processor B and download all this data to explore on your own. And don’t forget, you’ve done all this on your own, without any coding or data science work whatsoever. Thanks, Pagos!
The BIN-Based Strategy
Organized in descending order of transaction count, the top 10 BINs processed through both processors have some overlap, but with some glaring differences in approval rate. Let’s look at that sample data:

Immediately, you identify two BINs for which the approval rate via Processor B is significantly higher than Processor A. BIN 222222 has a 76% approval rate when processed through Processor A and a 92% approval rate through Processor B. Similarly, BIN 777777 has an approval rate of 70% for Processor A and a 97% approval rate through Processor B. Given these values, you have a real opportunity here for improving your overall card approval rate—and bottom line—by sending more transactions associated with both BINS through Processor B over Processor A.
You could perform a similar analysis across all of your BINs and design routing rules to push BINs with the higher success rates to the associated processor.
Side Note: For the purposes of A/B testing, we recommend only pushing a portion of a BIN’s transactions to the processor with the higher approval rate. This allows you to continue to compare transaction approval rates for those BINs across processors side-by-side to ensure the witnessed trend wasn’t a temporary occurrence.
Ongoing BIN-Based Monitoring
So far, you’ve used BINs to analyze your data in digestible segments and designed a routing strategy around those same BINs. Next, you want to continuously monitor these BINs to dictate how your routing strategy evolves moving forward. As with all things in the payments world, changes will happen over time and some BINs that previously had higher success rates through one processor may suddenly do better through another. You can create custom dashboards in Pagos to monitor the approval rates by processor for all the BINs you designed routing rules around or even set up alerts to notify you should approval rates for specific BINs drop below expected levels.
Since Pagos also ingests your refund and chargeback data, you can even start researching potential root causes around particular issuer and BIN performance over longer periods of time, and further modify your routing or processing strategy. Similarly, in instances where approval rates through Processor A and Processor B reach an equal level, you can use Pagos to compare the cost of processing certain BINs through each processor and make additional changes. We will cover more of this in future posts!
Now It’s Your Turn
Integrating BINs into any payments data analysis can unveil new strategies for enhancing approval rates and optimizing revenue. By leveraging BIN data, you can segment your customer base effectively, enabling you to make informed decisions around transaction routing, fraud rules, and more. If you’re looking for an easy, no-code solution for monitoring all your payments data in one place, look no further than Pagos. We have the payment intelligence and observability tools you need to optimize your processing strategies. Contact us to get started today!
Given the recent explosion in online payments, available payment methods, and payment routing options, companies have a lot to think about when it comes to processing transactions. One such challenge—and opportunity—is deciding which processor to send any given transaction to. While theoretically a payment sent to any processor should see the same result (e.g. success or failure), the complexity of the payments ecosystem and the sheer number of players involved means that’s not always the case. In reality, it’s easy for two processors to diverge in performance due to data quality, compliance updates, fraud rules, integration setup, and even customer mix changes.
In this blog post, we’ll take you through an example of how you can make data-driven decisions around transaction routing using payment card Bank Identification Numbers (BINs). This complete use case explores incorporating BIN data not just into your processing/routing analysis, but into the executed strategy and ongoing monitoring of the results. Being data driven means you should baseline your performance in a normalized way, establish a hypothesis to test, and then track the performance of a given change; in this case we cover routing, but a similar approach can be used for chargeback metrics, cost metrics, and more. Let’s get started!
Note: While this example was written using real data, all data and BINs have been changed or anonymized for the purpose of this story. You can also assume here that the transaction volume distributed across the two processors and BIN is sufficient for a comparison, and that other related metrics like chargeback rates and refund rates are sufficiently similar.
Why BINs?
In previous blog posts, we’ve discussed the importance of integrating bank identification numbers (BINs) into your payments analysis, even going so far as to cite BIN data as a key part of any payments optimization strategy. When it comes to learning who your customers are, where they’re located, and what their preferences are, you can’t do much better than BIN data. Similarly, BINs allow you to divide your customer base up into logical segments for making informed decisions around processing, fraud analysis, tokenization, and more. In this post, we’ll use BINs as a segmentation tool for transaction routing.
The BIN-Based Analysis
To make this post more fun, we’re going to put you in the role of the payments manager at a mid-sized business. Imagine your business processes transactions in the United States through two processors. Your current routing strategy is to route roughly 25% of your transactions through Processor A and 75% through Processor B. Your overall approval rate across all your card transaction volume is fair—around 86%—but you want to improve it to increase your revenue. You’re specifically interested in how you might be able to increase your overall approval rate by being more strategic with your transaction routing.
To better understand your approval rate (and how to improve it), you start a deep dive analysis with Pagos. Because Pagos ingests all your data from both your processors and aggregates it together into a single, harmonized data set, you can see all your transaction data in one place, broken down by whatever parameters matter to you most. For this particular investigation, you’re interested in seeing your approval rate broken down by BIN for both processors. In other words, you want to see if some BINs perform better with one payment processor over the other; if so, you can design routing rules to send transactions made with certain BIN ranges to the processor where it’s most likely to succeed!
For this analysis, you filter your data from the last six months to only see transactions routed through Processor A, broken down by BIN. And for each BIN, you want to see attempted vs. approved transaction counts and value, alongside the approval rate. You do the same for transactions from Processor B and download all this data to explore on your own. And don’t forget, you’ve done all this on your own, without any coding or data science work whatsoever. Thanks, Pagos!
The BIN-Based Strategy
Organized in descending order of transaction count, the top 10 BINs processed through both processors have some overlap, but with some glaring differences in approval rate. Let’s look at that sample data:

Immediately, you identify two BINs for which the approval rate via Processor B is significantly higher than Processor A. BIN 222222 has a 76% approval rate when processed through Processor A and a 92% approval rate through Processor B. Similarly, BIN 777777 has an approval rate of 70% for Processor A and a 97% approval rate through Processor B. Given these values, you have a real opportunity here for improving your overall card approval rate—and bottom line—by sending more transactions associated with both BINS through Processor B over Processor A.
You could perform a similar analysis across all of your BINs and design routing rules to push BINs with the higher success rates to the associated processor.
Side Note: For the purposes of A/B testing, we recommend only pushing a portion of a BIN’s transactions to the processor with the higher approval rate. This allows you to continue to compare transaction approval rates for those BINs across processors side-by-side to ensure the witnessed trend wasn’t a temporary occurrence.
Ongoing BIN-Based Monitoring
So far, you’ve used BINs to analyze your data in digestible segments and designed a routing strategy around those same BINs. Next, you want to continuously monitor these BINs to dictate how your routing strategy evolves moving forward. As with all things in the payments world, changes will happen over time and some BINs that previously had higher success rates through one processor may suddenly do better through another. You can create custom dashboards in Pagos to monitor the approval rates by processor for all the BINs you designed routing rules around or even set up alerts to notify you should approval rates for specific BINs drop below expected levels.
Since Pagos also ingests your refund and chargeback data, you can even start researching potential root causes around particular issuer and BIN performance over longer periods of time, and further modify your routing or processing strategy. Similarly, in instances where approval rates through Processor A and Processor B reach an equal level, you can use Pagos to compare the cost of processing certain BINs through each processor and make additional changes. We will cover more of this in future posts!
Now It’s Your Turn
Integrating BINs into any payments data analysis can unveil new strategies for enhancing approval rates and optimizing revenue. By leveraging BIN data, you can segment your customer base effectively, enabling you to make informed decisions around transaction routing, fraud rules, and more. If you’re looking for an easy, no-code solution for monitoring all your payments data in one place, look no further than Pagos. We have the payment intelligence and observability tools you need to optimize your processing strategies. Contact us to get started today!
Given the recent explosion in online payments, available payment methods, and payment routing options, companies have a lot to think about when it comes to processing transactions. One such challenge—and opportunity—is deciding which processor to send any given transaction to. While theoretically a payment sent to any processor should see the same result (e.g. success or failure), the complexity of the payments ecosystem and the sheer number of players involved means that’s not always the case. In reality, it’s easy for two processors to diverge in performance due to data quality, compliance updates, fraud rules, integration setup, and even customer mix changes.
In this blog post, we’ll take you through an example of how you can make data-driven decisions around transaction routing using payment card Bank Identification Numbers (BINs). This complete use case explores incorporating BIN data not just into your processing/routing analysis, but into the executed strategy and ongoing monitoring of the results. Being data driven means you should baseline your performance in a normalized way, establish a hypothesis to test, and then track the performance of a given change; in this case we cover routing, but a similar approach can be used for chargeback metrics, cost metrics, and more. Let’s get started!
Note: While this example was written using real data, all data and BINs have been changed or anonymized for the purpose of this story. You can also assume here that the transaction volume distributed across the two processors and BIN is sufficient for a comparison, and that other related metrics like chargeback rates and refund rates are sufficiently similar.
Why BINs?
In previous blog posts, we’ve discussed the importance of integrating bank identification numbers (BINs) into your payments analysis, even going so far as to cite BIN data as a key part of any payments optimization strategy. When it comes to learning who your customers are, where they’re located, and what their preferences are, you can’t do much better than BIN data. Similarly, BINs allow you to divide your customer base up into logical segments for making informed decisions around processing, fraud analysis, tokenization, and more. In this post, we’ll use BINs as a segmentation tool for transaction routing.
The BIN-Based Analysis
To make this post more fun, we’re going to put you in the role of the payments manager at a mid-sized business. Imagine your business processes transactions in the United States through two processors. Your current routing strategy is to route roughly 25% of your transactions through Processor A and 75% through Processor B. Your overall approval rate across all your card transaction volume is fair—around 86%—but you want to improve it to increase your revenue. You’re specifically interested in how you might be able to increase your overall approval rate by being more strategic with your transaction routing.
To better understand your approval rate (and how to improve it), you start a deep dive analysis with Pagos. Because Pagos ingests all your data from both your processors and aggregates it together into a single, harmonized data set, you can see all your transaction data in one place, broken down by whatever parameters matter to you most. For this particular investigation, you’re interested in seeing your approval rate broken down by BIN for both processors. In other words, you want to see if some BINs perform better with one payment processor over the other; if so, you can design routing rules to send transactions made with certain BIN ranges to the processor where it’s most likely to succeed!
For this analysis, you filter your data from the last six months to only see transactions routed through Processor A, broken down by BIN. And for each BIN, you want to see attempted vs. approved transaction counts and value, alongside the approval rate. You do the same for transactions from Processor B and download all this data to explore on your own. And don’t forget, you’ve done all this on your own, without any coding or data science work whatsoever. Thanks, Pagos!
The BIN-Based Strategy
Organized in descending order of transaction count, the top 10 BINs processed through both processors have some overlap, but with some glaring differences in approval rate. Let’s look at that sample data:

Immediately, you identify two BINs for which the approval rate via Processor B is significantly higher than Processor A. BIN 222222 has a 76% approval rate when processed through Processor A and a 92% approval rate through Processor B. Similarly, BIN 777777 has an approval rate of 70% for Processor A and a 97% approval rate through Processor B. Given these values, you have a real opportunity here for improving your overall card approval rate—and bottom line—by sending more transactions associated with both BINS through Processor B over Processor A.
You could perform a similar analysis across all of your BINs and design routing rules to push BINs with the higher success rates to the associated processor.
Side Note: For the purposes of A/B testing, we recommend only pushing a portion of a BIN’s transactions to the processor with the higher approval rate. This allows you to continue to compare transaction approval rates for those BINs across processors side-by-side to ensure the witnessed trend wasn’t a temporary occurrence.
Ongoing BIN-Based Monitoring
So far, you’ve used BINs to analyze your data in digestible segments and designed a routing strategy around those same BINs. Next, you want to continuously monitor these BINs to dictate how your routing strategy evolves moving forward. As with all things in the payments world, changes will happen over time and some BINs that previously had higher success rates through one processor may suddenly do better through another. You can create custom dashboards in Pagos to monitor the approval rates by processor for all the BINs you designed routing rules around or even set up alerts to notify you should approval rates for specific BINs drop below expected levels.
Since Pagos also ingests your refund and chargeback data, you can even start researching potential root causes around particular issuer and BIN performance over longer periods of time, and further modify your routing or processing strategy. Similarly, in instances where approval rates through Processor A and Processor B reach an equal level, you can use Pagos to compare the cost of processing certain BINs through each processor and make additional changes. We will cover more of this in future posts!
Now It’s Your Turn
Integrating BINs into any payments data analysis can unveil new strategies for enhancing approval rates and optimizing revenue. By leveraging BIN data, you can segment your customer base effectively, enabling you to make informed decisions around transaction routing, fraud rules, and more. If you’re looking for an easy, no-code solution for monitoring all your payments data in one place, look no further than Pagos. We have the payment intelligence and observability tools you need to optimize your processing strategies. Contact us to get started today!
Share this Blog Post
Share this Blog Post
Latest Blog Posts

Turning Payments Data into Realistic Cost Savings
Turning Payments Data into Realistic Cost Savings
Turning Payments Data into Realistic Cost Savings

Payments Metrics: A Delicate Balance
Payments Metrics: A Delicate Balance
Payments Metrics: A Delicate Balance

Hidden Insights Available Through Network Tokenization
Hidden Insights Available Through Network Tokenization
Hidden Insights Available Through Network Tokenization
Subscribe to our Blog
Subscribe to
our Blog
Subscribe to our Blog
By submitting, you are providing your consent for future communication in accordance with the Pagos Privacy Policy.